Recent Blog Entries
Share
How to Identify and Prevent Batch Effects in Longitudinal Flow Cytometry Research Studies
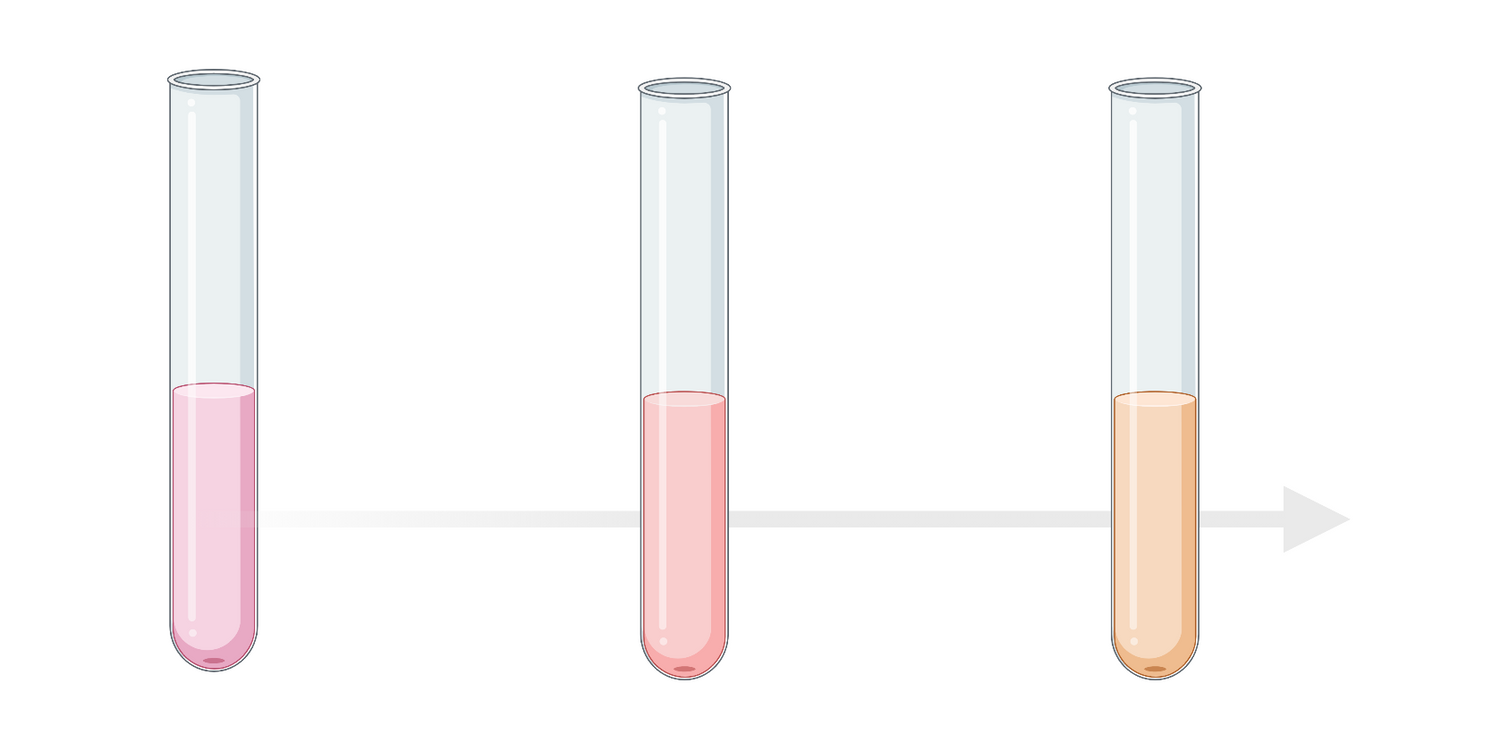
This blog was prepared and written by Geoff Kraker, Technical Application Specialist - Software Platforms, Cytek Biosciences
When planning a long-term study or clinical trial that includes collecting and analyzing samples on a flow cytometer across weeks, months, or years, what are some steps you can take to mitigate the impact of batch effects on the resulting analysis? Here we describe batch effects and how to identify them in your data, tips to prevent them, and possible fixes.
What are batch effects?
A batch effect is a measurement that has a qualitatively different behavior across experimental conditions while being unrelated to the scientific variables in the study. Some real-life examples of what can cause batch effects include:
- Running out of a tandem conjugated antibody in the middle of the study and using a replacement bottle from a different lot. This new lot may have a different donor to acceptor ratio, causing the signal to be brighter or dimmer for the same number of targets in the cell. Batch variation between antibody-fluor conjugates can introduce significant variation in your data, particularly when using tandem dyes. Further, dye intensity can vary between lots causing a brighter or dimmer signal from the same volume highlighting the importance of antibody titration.
- Two different technicians prepare samples and they each pipette consistently but differently, leading to staining differences. Both technicians follow the protocol as written, but small unwritten details can contribute to differences in the resulting data.
- Not letting the instrument warm up before starting acquisition/QC
- Inconsistent storage conditions between groups of samples, e.g., one freezer failing while another stays at the correct temperature.
- Replacement of a laser or detector module during the study.
- Differences in staining protocol, including changing buffers, reagents, incubation times, or the number of washes.
- Unintentional differences in sample collection, e.g., using different anticoagulants or letting one sample sit on the bench longer before processing.
- Treating the samples differently in transit or storage.
- Changing the acquisition settings between samples.
- Leaving an antibody out of the master-mix and then staining the samples.
- Having "healthy" controls exhibit higher rates of viral challenge in a typical winter than in summer or higher levels of stimulation during a specific allergy season than winter.
These are just a few examples, and while there are almost limitless sources of batch effects, it's possible to eliminate the most likely sources through diligent experimental planning.
Why do batch effects matter?
Batch effects matter because they can blunt the findings of a study, confound the possible conclusions, and even worse, potentially supplant the presumed experimental source of change as the main conclusion of the study [1]. All these sources often present themselves by proxy, either by experimental group or processing date. This means that the signal across time will change, and it will take some investigating to uncover the real source of the variation between batches as "time between batches" alone is very often not the root cause of the issue.
One of the most simple and effective ways to combat batch effects is to include a "bridge", "anchor", or "validation" sample in each batch. The goal is to have a consistent sample present in each batch so batches can be compared and any shift in the results can be visualized and quantified. How to accomplish this will be addressed later, but it bears emphasizing that this is a simple and effective measure that should be employed in most, if not all, longitudinal studies.
How do you check your data for batch effects?
The first step is to determine if there are batch effects in the data set. There are several ways to do this – ranging from simple qualitative approaches to algorithmic-driven evaluation. Here we cover a range of choices.
- It is sometimes possible to find batch effects by plotting histograms of single channels overlaid by batch and then checking for grouping or splitting of the samples. This is most effective with constitutively expressed lineage markers that are not expected to change with experimental conditions e.g. CD45, CD3, CD19, or CD14.
- A more advanced approach is to plot channels from an aliquot of the same "bridge" or "anchor" sample in each batch on a Levy-Jennings chart. If a batch effect skews the channels of interest up or down, this will be visible on the LJ chart. These approaches are more effective if the batch effect is large, but small changes can still sometimes be detected qualitatively. [2]
- Dimensionality Reduction algorithms are often part of analysis workflows and can act as a relatively simple way to check for batch effects, even if the changes are too small to spot in individual channels. When batch effects are present in an experiment and alter the surface marker expression used in generating the tSNE or UMAP variables, the result can look like this:
This is data from three samples acquired over two months and run on the same instrument. Green and Orange were run just a few days apart, while Blue was run7 weeks later. If most, or all, of the populations appear in a similar but not identical location in the plot, there's a chance that this shift is caused by a batch effect. This shift could also be due to biological variation between samples, however that may appear as a difference in only a few of the islands.
This next example contains four files overlaid from a different study with very little change in cluster positions. All four samples were stained and run on the same instrument on the same day. The arrow shows a good example of the difference in population abundance across samples - the middle of the island isn't shifting; the blue sample just has fewer events of that particular phenotype than the orange sample. As above, a batch effect can be especially obvious on a dimensionality reduction plot if samples from the same batch are concatenated (real or virtually) and displayed overlaid on other batches. If the difference between batches needs to be quantified, it's possible to calculate the Jensen-Shannon Divergence of the UMAP/tSNE parameters between samples/batches to get a quantitative comparison of each batch’s island positions. The Jensen-Shannon (JS) divergence is an information theory-based, symmetric measure of the similarity between two probability distributions [3] [4] [5].
- A more quantitative but complication approach involved using algorithms like Harmony [6] or iMUBAC [7] to identify and correct batch effects. Both solutions require the user to do at least some, and potentially substantial preparation of the files before calculation. The benefits of scalable, automated, and unsupervised analysis shouldn't be understated.
How do you prevent batch effects?
The best way to fix a batch effect is to stop it before it becomes a problem – an ounce of prevention is worth a pound of cure as the saying goes. It's not possible to completely eliminate all sources of batch variations but implementing a few measures at the start of a study can save time and trouble later when analyzing the data.
Start with experiment planning and control over study execution. Make sure everyone involved with the study (physicians, clinical coordinators, techs, shared resource facilities, etc.) are all on the same page when it comes to sample timing and standard operating procedures. Although it sounds elementary, making sure things like keeping similar timing from bedside to bench and collecting blood in the same type of anticoagulant are vital to the downstream quality of the samples.
It is also incredibly important to ensure that all reagents are titrated correctly for the number and type of cells expected in the samples. If the antibodies are titrated on 100,000 cells per test, and a patient sample comes in with 5,000,000 cells, the sample will likely be understained. It may be worth performing a cell count and then normalizing cell counts per sample to ensure even staining.
A relatively simple way to address batch effects is to ensure a specific peak in a bead control falls near (or in) the same channel on the cytometer before experimental sample acquisition. The overarching goal is to take a particle with a fixed fluorescence and make sure it is detected at the same level before each batch is acquired, leading to consistency across batches from a detection standpoint. Many cytometers including the Cytek® Aurora and Cytek® Northern Lights™ systems have built-in QC programs with this functionality. While this helps control for day-to-day instrument variation, it won't reduce variability in sample preparation or staining. Since changes can happen over the course of the day as well, it is best practice to run an MFI target value test before every sample in the study to verify the cytometer is detecting your channel/s of interest in the correct range.
Another simple way to combat batch effects is to make sure experimental groups are mixed across acquisition sessions. Acquiring all control samples on one day, all group 1 samples the next day, and then all group 2 samples on the final day is a great way of introducing batch effects to an otherwise well controlled experiment. If samples are banked, randomizing which samples are included in which acquisition session is a good way to minimize batch effects. Other experimental design suggestions and best practices are available in Thomas Liechti’s CYTO U Lecture from October 2020. [8]
To eliminate batch effects from the staining and acquisition process, fluorescent cell barcoding can be employed. This technique involves uniquely labeling each sample with a set of fluorescent tags, mixing the samples together, staining them all in a single tube, washing, and then acquiring the single tube that contains all samples (or a batch of samples). After acquisition, the data is then de-barcoded by plotting the barcoding channels against each other and drawing gates around each "population" which equates to each original sample.

These papers by Peter Krutzik et al. [9] and David Earl et al. [10] offer some technical perspective and guidance on accomplishing this technically challenging task. If performed effectively, this allows a group of samples to be stained and run under the exact same conditions, eliminating batch effects. Differences in sample collection and storage may still be visible, but barcoding will go a long way towards reducing the effects of differential sample prep and acquisition.
When undertaking a longitudinal study using spectral cytometry, some consideration should be given to what kind of reference controls will be used (whether beads or cells) - either collecting a new set of controls for each batch or sample, or collecting a 'gold-standard' set at the beginning. Choosing correctly can also aid in preventing batch effects. Using one set of initial reference controls might be indicated if the reagents are known to be stable, the samples in the study are well characterized and persistent, and there is an increased risk of technical preparation errors expected during the study. Per batch sets of reference controls may be indicated if the batch number is low, reagent stability is in question, the samples (and their autofluorescence) are poorly characterized, or there is no question that the experiments will be executed with a high technical proficiency.
How do you fix batch effects?
Like we mentioned above, one commonly used method to both identify and (if necessary) fix batch effects is the inclusion of a "bridge", "anchor", or "validation" sample in each batch. The goal is to have a consistent sample present in each batch so batches can be compared and any shift in the results can be visualized and quantified. This can be achieved in several ways, but commonly investigators working with PBMCs will aliquot and freeze a leukopak or some similar large single source of cells and then for each batch of the study, remove a vial and prep the cells alongside the experimental samples. While not ideal, even if the assay/trial in question only involves fresh samples, the bridge sample must only match itself across batches so may be a suitable method for tracking changes over time. While generally effective for its stated purpose, this method isn't suitable for all situations. Sometimes a cell population of interest is rare enough that including enough cells for each batch from a single source can be difficult, or the antigen of interest might disappear with freeze/thaw processing. In this case, a lyophilized cell control product can be a solution - regardless, choosing a control sample that allows each channel to be tracked in some capacity is essential for the success of this method.
Once these bridge samples are acquired, they can be examined across time in a Levy-Jennings plot to look for changes. They can also be used in algorithms like Harmony [6], cytoNorm [12], or iMUBAC [7] as a guide to which all the experimental samples can be normalized against. These tools will work on any cytometry data, including that generated by the Cytek® Aurora or Northern Lights™ systems.
Looking forward, there will inevitably be newer and more innovative algorithms and strategies for addressing batch effects in longitudinal experiments but in the meantime, with a little planning and the techniques listed above, you should be on your way towards cleaner and more reliable longitudinal data.
References
- Ransohoff DF. Lessons from controversy: ovarian cancer screening and serum proteomics. J Natl Cancer Inst. 2005 Feb 16;97(4):315-9. doi: 10.1093/jnci/dji054. PMID: 15713968.
- Levy-Jennings plot https://www.bangslabs.com/applications/flow-cytometry/blog/daily-monitoring-assess-instrument-performance
- Jensen-Shannon Divergence on two-dimensional maps. CytUtils quality controls and reproducibility utilities. Ichann School of Medicine Mt Sinai Hospital Immune Monitoring Core https://rdrr.io/github/ismmshimc/cytutils/man/calculate2dJsDivergence.html
- Amir, El-ad David, et al. "ViSNE enables visualization of high dimensional single-cell data and reveals phenotypic heterogeneity of leukemia." Nature Biotechnology, vol. 31, 19 May 2013, pp. 545-52, https://doi.org/10.1038/nbt.2594.
- Jensen-Shannon divergence supplementary information from [2] https://static-content.springer.com/esm/art%3A10.1038%2Fnbt.2594/MediaObjects/41587_2013_BFnbt2594_MOESM18_ESM.pdf
- Korsunsky, I., Millard, N., Fan, J et al.Fast, sensitive and accurate integration of single-cell data with Harmony. Nat Methods 16, 1289-1296 (2019).
- Ogishi, M., Yang, R., Gruber, C., et al, Multi-batch cytometry data integration for optimal immunophenotyping. the Journal of Immunology November 23, 2020, ji2000854; DOI: 10.4049/jimmunnol.2000854
- Liechti, T. Experimental Design and Quality Control for High-Dimensional Human Immunophenotyping Studies in Large Cohorts 10/2020 [Online] https://learning.isac-net.org/products/experimental-design-and-quality-control-for-high-dimensional-human-immunophenotyping-studies-in-large-cohorts
- Krutzik PO, Clutter MR, Trejo A, Nolan GP. Fluorescent cell barcoding for multiplex flow cytometry. Curr Protoc Cytom. 2011;Chapter 6:Unit-6.31. doi:10.1002/0471142956.cy0631s55
- Earl, D.C., Ferrell, P., Leelatian, N. et al.Discovery of human cell selective effector molecules using single cell multiplexed activity metabolomics. Nat Commun 9, 39 (2018). https://doi.org/10.1038/s41467-017-02470-8
- Van Gassen, S., Gaudilliere, B., Angst, M.S., Saeys, Y. and Aghaeepour, N. (2020), CytoNorm: A Normalization Algorithm for Cytometry Data. Cytometry, 97: 268-278. https://doi.org/10.1002/cyto.a.23904
Cover image created with Biorender.com